Nishita Belur L&S Biological Sciences
Computational mechanisms underlying extinction learning and relapse
In patients with Alcohol Use Disorder (AUD), repeated activation of dopaminergic neurons facilitates associative learning, as patients associate reward-predictive contexts with reward delivery, in this case, drug administration. AUD is difficult to treat because of the possibility of relapse, which can be modeled as reacquisition of a previously extinguished behavior in a reward learning context. Our focus is the “savings effect”, in which some “original memory” is “saved”, making reacquisition much faster than initial acquisition. The dominant model of reward learning, the Temporal Difference Reinforcement Learning model, relies on prospective predictions of rewards from cues and does not explain this phenomenon. Thus, Jeong et al. proposed a new model, Adjusted Net Contingency for Causal Relations (ANCCR), which hypothesized that inferring causal cues of reward delivery retrospectively could be the “original memory.” This summer, we will investigate ANCCR predictions for behavior reacquisition in a classical conditioning task for a mice AUD phenotype. Understanding how memory factors into behavioral extinction is uniquely key for AUD, as it can help prevent relapse long-term.
Message To Sponsor
Thank you so much for funding my research this summer. I made mistakes, but I learned a lot about conducting research and resilience. I saw my skills tangibly improve through the summer: Your support helped me dedicate my full time to the project. I hope to pursue graduate study as an MD PhD and continue to research the neural mechanisms behind addiction.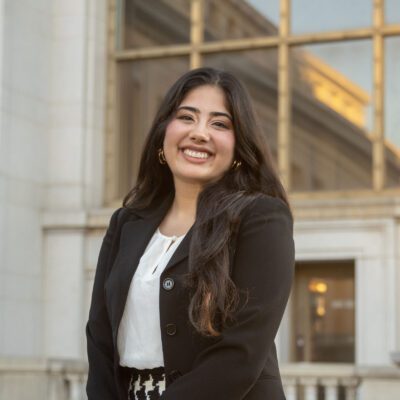