Justin Uang Rose Hills
Textured-Object Detection and Robotic Perception
Perception, or the ability to perceive the world, is one of the remaining missing components for allowing robots to enter our daily environments. However, its current state-of-the-art performance is far from accurate. This summer, I will explore several machine learning techniques for improving perception. For instance, I will use distance metric learning to improve upon the euclidean distance that is widely used for comparing data points. Preliminary results show that algorithms such as Large Margin Nearest Neighbor can greatly improve k-nearest-neighbor performance for SIFT features, improving perception performance. I also plan to use structured data with unsupervised feature learning to improve the feature descriptors used in perception systems. Namely, the features that we hope to learn will capture local depth structure, albedo, and lighting.
Message To Sponsor
I am grateful to the Rose Hills Foundation for the rare opportunity to focus on one problem for an extended period of time, without the distractions of school and other activities. My research particularly excites me because I believe in the potential of computer perception to permeate and benefit society. Finally, I think the chance to be acquainted with graduate school life will be an invaluable experience for helping me decide what paths to take in the future.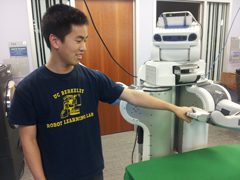