Renzo Soatto Rose Hills
WH Regularization in Deep Learning Models of Biological Sequences
Throughout this summer, I will be designing and implementing a novel regularization technique to improve the performance of deep learning models for predicting properties of biological sequences, such as proteins or regulatory DNA.
Predicting properties of biological sequences has many applications, ranging from understanding disease mechanisms to designing new therapeutics. However, deep learning struggles with this task because most data sets are small and/or extremely noisy, due to the challenges of conducting the required wet-lab experiments. Without large amounts of high-quality data, models need to have the right inductive biases, which can be achieved through regularization, in order to prevent overfitting to the training data.
In this project, I will leverage the Walsh-Hadamard transform, a technique from signal processing theory that allows for a highly interpretable form of regularization for biological sequences, to develop novel regularization strategies. If successful, these strategies could also be used to improve applications of machine learning to predicting properties of non-biological discrete sequences, for example in meteorology, psychology, or security.
Message To Sponsor
To all those who are supporting my research this summer, I hope this message finds you well. I want to take a moment to express my heartfelt gratitude for your support; your contribution will both allow me to pursue my academic interests this summer and enable me to gain invaluable experience and skills that will benefit me throughout my career. I am honored to be the recipient of your support, and I promise to put it to good use. Thank you again for your kindness and generosity.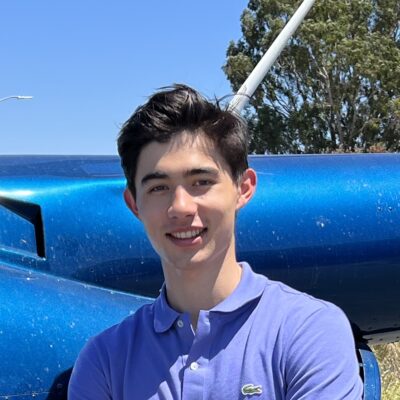