Max Litster L&S Sciences
The Inferred Reader: Natural Language Processing for Narrative Understanding
Understanding literary narratives requires a strong grasp of the underlying language; unlike text drawn from Wikipedia, social media, or the news, literary prose is ripe with embellished descriptions and figurative language. Plot points are revealed in the form of nuanced events rather than explicit recollections: we read about a character’s ‘final breath’ rather than their death. The success of attention-based neural networks on an assortment of semantic inference and interpretation tasks suggests that they provide an effective model of human language, but a noticeable drop in performance when applied to literary data has inspired a call for domain-specific systems. This work draws on equal parts natural language processing research and literary theory to develop such ‘born-literary’ NLP models, designed specifically for the task of narrative understanding. If successful in predicting plot outcomes, the representations learned by these models may capture some of the latent narrative structures that encode storytelling devices like foreshadowing, offering a new, statistical perspective towards the elements of literature that make the medium so engaging.
Message To Sponsor
I am very grateful to my donor for allowing me to pursue this opportunity. I have always loved literature and computer science, and I'm thrilled to finally be able to combine my passions in a meaningful way. I'm very interested in pursuing grad school, so I'm very grateful for the chance to gain independent research experience beforehand.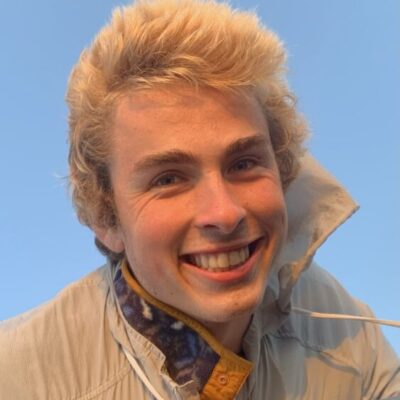