Minjune Hwang L&S Sciences
Differential Model Predictive Control for Autonomous Driving in Under-structured Traffic Environment
In recent years, rapid growth in self-driving vehicles was largely enabled by breakthroughs in computer vision and reinforcement learning (RL). Current research focuses on applications of RL-based, model-free methods through simulation rather than classical model-based optimization. However, model-free algorithms often fail to generalize beyond the environment in which it was trained. For instance, self-driving cars trained in a regular urban environment will suffer to drive safely and efficiently in road environments in developing countries where traffic signals do not exist, roads are under-structured, and drivers drive under implicit rules. Subsequently, for autonomous vehicles, navigating safely but efficiently in under-structured, under-regulated road environment has remained as a major challenge. Thus, deployment of self-driving cars in developing countries is challenging, as it is hard for RL models to find the sweet spot between ensuring safety and avoiding congestion or delays, in such a traffic environment, with insufficient training data and poor generalization.
With a traffic trajectory dataset in under-regulated local traffic (intersections and highways) that my team collected, I aim to model human drivers behaviors and compute safe and efficient trajectory planner in such traffic environments by using differential Model Predictive Control (MPC), a state-of-the-art model-based controller, to both learn dynamics and plan trajectories by differentiating optimization problems in continuous space, which will mitigate problems in current model-based and model-free algorithms, given real-world trajectory data.
Message To Sponsor
I am very grateful to Chen Fund, for providing this precious opportunity that allowed me to conduct my research project over the summer. Through this program, I was able to pursue my interest in computer vision and reinforcement learning, helping me to grow as a person. Thanks to the support of SURF program, I was also able to interact with my mentors, advisors, and other peers in the program to learn not only about how to conduct a research project and effectively present my project, but also about other intriguing projects by peers in the program. This experience really strengthened my ability as a researcher, and furthered my interest in machine learning field. Thank you again for the generosity of Chen Fund and the support of SURF program for allowing me to pursue my research project over the summer.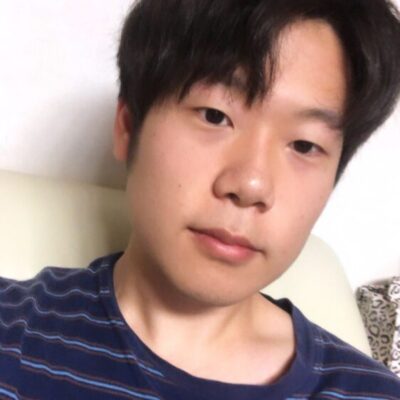