Nathan Cheng L&S Sciences
Analysis of Matrix Multiplication Complexity Using Properties of Tensors
Matrix multiplication is one of the most foundational mathematical operations, and has deep connections to many areas of math, including algebra, geometry, and combinatorics. There is huge incentive to improve the speed of matrix multiplication as well as understand the inherent bounds on its complexity, due to its importance in applied mathematics and the computational sciences.
Many of the questions concerning the complexity of matrix multiplication are still unsettled, namely: how can we make it faster and how fast can it go? It is thought that intrinsic mathematical properties of matrices constrain the algorithmic possibilities for operations on them. This is the thesis of algebraic complexity theory. Such properties include the dimensions of certain functions of matrices, many of which are not known. The purpose of this project is to perform a systematic application of known techniques from algebraic complexity theory to understand simple cases which are still poorly understood (which to date has not been performed). We hope to use techniques such as border rank bounds, basis transformations, tensor-flattening approaches, and group-theoretic algorithms, and demonstrate either the power or the limitations of such techniques.
Message To Sponsor
I would like to thank the Sherrill fund for sponsoring our team's efforts this summer. Personally, this experience gave me a chance to develop my skills as an independent researcher and also helped me define and sharpen my interests. As I look towards graduate school and my future in general, I think I have a better idea of the kind of research, knowledge, and work I want to pursue after this summer. Along the way, I was able to learn a lot about mathematics and mathematical problem solving, as well as get a taste for a very active and serious field of mathematics. This experience was made possible by the efforts of the SURF foundation, our mentor, and our donor. Many thanks to all.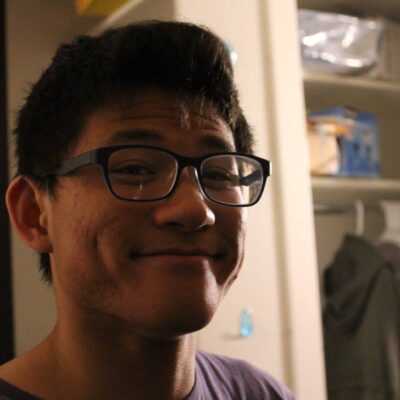