Haley Keglovits L&S Sciences
Computational Modeling of Reinforcement Learning and Working Memory Systems
Learning is paramount to human success, but the complex processes that underlie it are poorly understood. While cognitive models adequately explain some learning mechanisms, much remains to be discovered about the interactions between different mechanisms. Reinforcement learning (RL) is a slow and robust process that relies on external rewards to guide behavior. Working memory (WM) is a system that can retain recently acquired information for short periods of time. While previous research has demonstrated that both systems contribute to learning, the two mechanisms have rarely been studied together. In this project, I seek to examine what factors may affect how we recruit WM vs. RL for learning. Specifically, I would like to understand how being able to easily label a stimulus modifies the balance of WM and RL for learning. I hope to contribute to our knowledge of learning disorders, best practices in education, and computational neuroscience by testing how the what of learning affects the how of learning.
Message To Sponsor
Thank you so much to the Pergo Fund for sponsoring my summer experience-- it has affected my life and understanding of the research process in amazing ways I couldn't have imagined coming in. Being able to work full time on my project not only allowed me the time to develop deeper questions and methods than I would have been able to during the school year, it also gave me a taste of what being a graduate student will be like. I learned so much about myself and my work style that I couldn't in just a traditional semester with classes going on, and it has improved both my approach to the research process and how I tackle projects inside and outside the lab. It is because of you I was able to explore my passion, and I am eternally grateful for the opportunity you provided. I know that this project is the foundation for more research in graduate school and beyond, all thanks to you.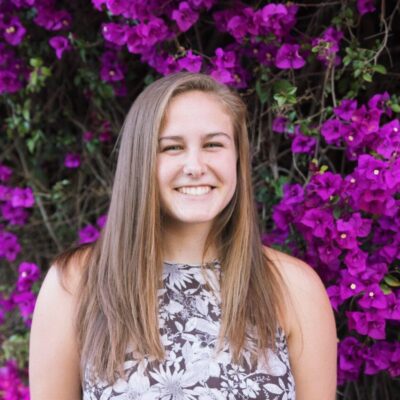