Hongli (Bob) Zhao L&S Sciences
Data Driven Approach to Uncertainty Quantification and Numerical Stochastic ODEs
From modeling cardiovascular circulation, to designing power grids or complex financial derivative products, the study of how noise influences a probabilistic system’s state is vital for successful financial, biological and physical models. Uncertainty quantification aims to explore how uncertainty is propagated throughout computational models via constitutive laws of dynamics. Building upon previous literature, I start by focusing on coupled stochastic balance laws on each edge of a network, whose numerical characterizations of the solution can be achieved with the PDF method. On the other hand, the derivations are problem dependent and at times present computational difficulties due to numerical instability. Therefore, the main focus of my project is to develop a general data-informed solver based on Newtonian relaxation, nonlinear multiple regression and neural network learning. The data-driven approach has potentially two implications in advancing the field: To provide an optimized alternative to Monte Carlo simulations; To design a strategy for analyzing large-scale hyperbolic systems whose coefficients are difficult to derive analytically. Extending the project, I seek to develop a solver library that adaptively chooses solution strategies based on characteristics of stochastic systems at hand.
Message To Sponsor
As an undergraduate student in Applied Math, I must say that this fellowship has provided me with the opportunity and tools to have an early start in professional level research in academia; throughout the summer, I had many relatable and invaluable experiences interacting with my collaborators and mentors, and accomplished novel results. I would like to sincerely thank the Anselm M&PS Fund for connecting young scholars with research opportunities, and supporting them on their early career in academia.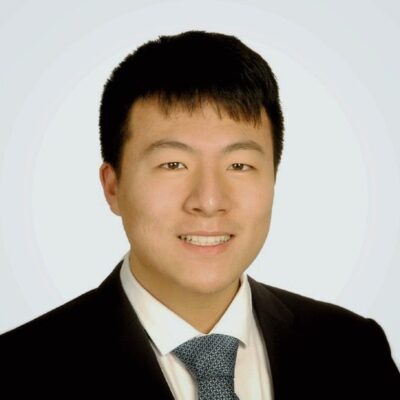