Sulaiman Alvi L&S Sciences
Quantum Machine Learning for High Energy Physics
The question I aim to answer this summer is whether quantum machine learning can provide an advantage over classical machine learning techniques for certain high energy physics applications. Analyzing data from particle collider experiments involves distinguishing signal events, which correspond to the particle physics of interest, from background events. Currently, classical machine learning techniques are used to tackle many of these classification problems. However, these techniques often require large amounts of data to classify with a high degree of certainty. In the growing area of anomaly-detection searches, datasets may be small. This can lead to poor classification performance of classical machine learning models. Several recent results have shown that quantum machine learning can provide an advantage for data-limited classification problems. However, these works have applied quantum machine learning to situations where classical machine learning techniques are already sufficient. Over this summer, I plan to directly test the possibility for a real quantum advantage by evaluating the success of
various parametrized quantum neural networks compared to a classical neural network
for quantum anomaly detection.
Message To Sponsor
I very much appreciate the financial support provided to me so that I can work on this project. Exploring different applications of quantum computing for physics is a subject I hope to one day pursue in graduate school. I am very grateful for the opportunity to gain research experience this summer working with leaders in the field.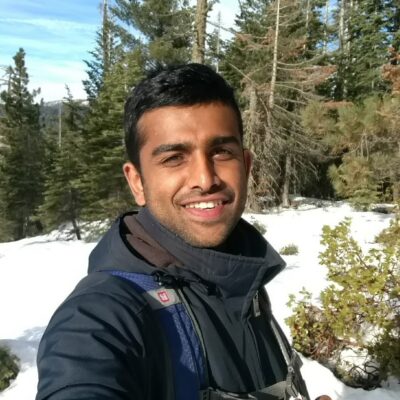