Doseok Austin Jang
Integrated Activation Maximization
Despite their popularity as predictive models, interpreting deep neural networks is still an open problem in machine learning. While local attribution techniques such as Integrated Gradients provide an importance map for individual input vectors, these techniques are unable to efficiently provide a global importance score over the entire input space. We introduce Integrated Activation Maximization (IAM), an iterative algorithm to jointly extract the activation maximizing pattern and a global attribution map for any node in a neural network faster than competing global attribution methods. IAM conducts a regularized gradient ascent to find the activation maximizing pattern for each node, while iteratively guiding the gradient ascent with on-the-fly feature importance estimates. IAM thus produces (1) a localized and focused optimal input pattern which emphasizes the features the model is most sensitive to, and (2) an attribution map that quantifies the importance of patterns in the optimal input.
Message To Sponsor
Thank you so much for helping support my research, it was a great experience that I have learned a lot from. Not having to worry as much about finances was a great boon to my experience, and I am sincerely thankful.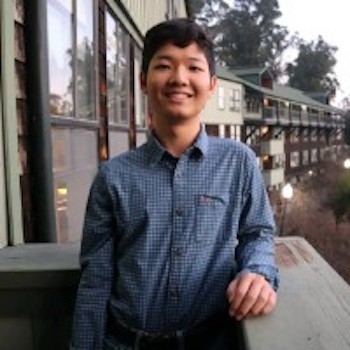